Implementing Artificial Intelligence in the Healthcare Field – some ethical concerns
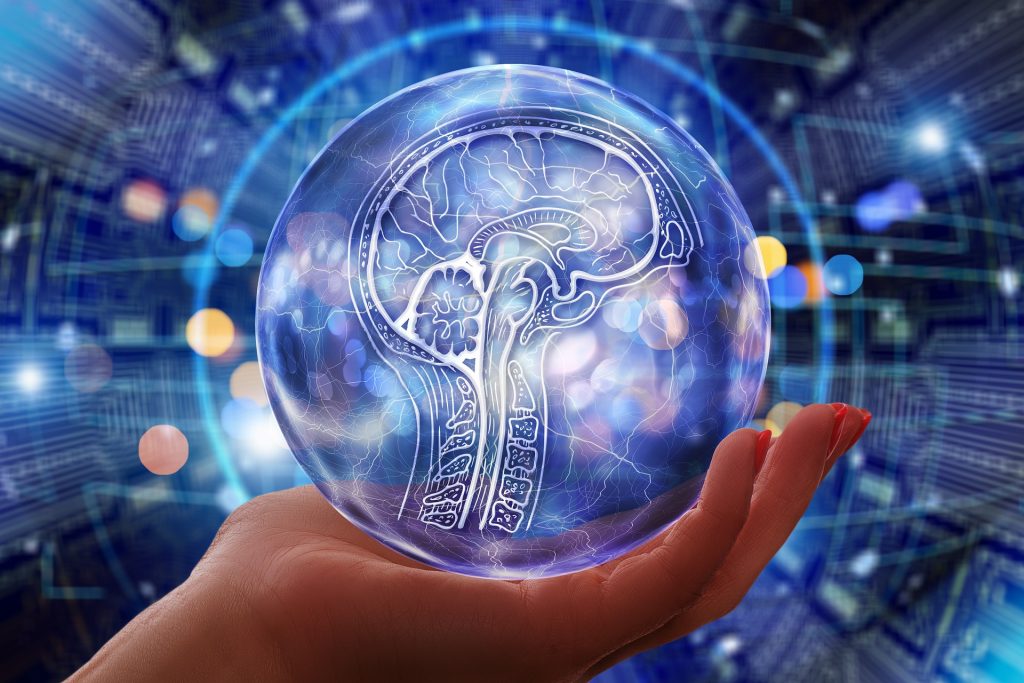
The development and implementation of Artificial Intelligence (AI)-based tools in the healthcare field seems to be continuously increasing. In fact, it would seem like there is no limit to human ingenuity when it comes to artificial intelligence.
The design and implementation of AI-based tools in healthcare, can be roughly classified into the following categories: a) AI in the service of medicine (health promoting technologies); b) AI in the service of medical practitioners (decision-supporting, prioritisation, and administrative-support tools); and c) AI in the service of social health justice (e.g., harnessing algorithms to remedy racial disparities in healthcare).
Admittedly, the line between the first two categories is somewhat blurry, as some applications, such as AI diagnostic tools, which substantially contribute to the promotion of patients’ health and medicine in general are, in fact, instrumental in supporting medical practitioners in their professional mission. I shall therefore, somewhat crudely, limit the second category of AI in the service of medical practitioners, to purely instrumental AI applications, mostly leaving applications that belong to such ‘hybrid’ category, with the group of AI tools in the service of medicine. I shall refrain from addressing the third category here, as it merits its own separate account.
AI in the service of medicine
Various imaginative AI-based tools are being developed to tackle medical challenges in various areas, such as IVF, mental health, oncology, and radiology. Seemingly, many of them are focused on bringing accurate, speedy and insightful diagnosis into the clinic.
The following are a few examples: some AI-based tools are used for prediction and diagnosis, inter alia for promoting preventive medicine. One such tool is aimed at identifying acute abnormalities such as cerebral bleeding and pulmonary embolism, and flagging them to radiographers. This tool allows for the prompt provision of care, as urgent circumstances reveal themselves through the application of AI technology.
Examples for other tools include: an AI-based tool that allows characterising, analysing, and predicting patient response to cancer treatment;a facial recognition technology assisting with diagnosis (e.g., recognising correlations between facial morphology and genetic conditions), patient identification, and medication adherence monitoring.
AI is also applied in clinical trials. In silico clinical trials, for example, use computer simulations and modelling in order to avoid the perils and inconveniences of conducting in vivo clinical trials; applying neural networks to predict adverse events or treatment compliance; and implementing models trained through machine learning, or deep learning, to better understand patient response differences. While still at their infancy, applying AI-based tools in clinical trials, also carry secondary benefits, as these become more flexible, speedier and significantly less costly. Also, in silico clinical trials will allow for a less cumbersome ethical review process. Where traditional ethical review boards are replaced with designated ethics committees with big data research expertise, approval efficiency can be significantly improved. Apparently, interventions involving AI, will have to adhere to specific reporting guidelines.
AI in the service of medical practitioners
AI-based tools can make a significant contribution to medical practitioners, by providing instructive outputs and by relieving overload in various direct and indirect ways.
Decision support systems can aid medical practitioners not only with reaching the right diagnosis in a short time, but also serve as prioritisation tools, assisting in workflow triage by flagging acute abnormalities. Take for example the ICU (intensive care unit) predictive tool, recently approved by the FDA. This AI-based technology, is designed to identify patients whose conditions are likely to deteriorate (as well as low-risk patients, unlikely to deteriorate). By using machine learning models, this AI tool enables better informed clinical decisions and early intervention, yielding improved outcomes and optimal ICU resource management.
Administrative-like tools, such as managing electronic health records (EHR), and freeing physicians from note-taking can be applied to relieve physicians from automation-prone tasks. These tolls carry a significant potential for reducing physicians’ burnout, allowing them instead to better communicate with patients and focus on actual healthcare delivery.
Ethical dilemmas
Alongside the hidden, and some already-manifested promises of AI for the betterment of healthcare, a rich variety of ethical dilemmas arise from the deployment of intelligent technology in the field. The development of AI tools for healthcare is rather unique, as these systems are trained and operating upon vast troves of personal, sensitive, medically confidential information (protected through various privacy and data security safeguards, according to jurisdiction-specific regulatory requirements). Also, the application of such AI tools is heavily built on trust, in a dual sense: it requires a leap of faith, both on part of the medical practitioner (confidence in the technology) and on part of the patient (confidence in the use of the technology, by the medical practitioner).
Universal concerns
Such concerns essentially reflect the inherent tension between human and smart machine. Or, in other words, they represent human apprehensions with respect to the adoption of AI-based technology into the field of healthcare, entrusted with human life, well-being, and reproduction.
Typical dilemmas include:
- How can AI be applied in ways that promote quality of care and curtail potentially disruptive effects?
- How can AI be integrated into healthcare in a way that reconciles its optimal potential with due safeguards for patient autonomy, safety and privacy?
AI ethics concerns
Various ethics concerns crop up where AI-based tools are being implemented in the field of healthcare. The following seem to be the most salient ones:
- Algorithmic bias. The source for such bias is twofold: 1) Data bias 2) Ill-diversified developers (inadvertently bringing inherent bias into data selection for the system’s training). Data bias in the context of healthcare, can be often attributed to historic selection bias, or inadequate and unfair representation of marginalised groups in the data. This is typically the result of decades-long underrepresentation of such groups in medical research, and lower healthcare consumption due to fundamental healthcare inequalities.
- Transparency. patients and research participants have a right to be informed that an AI tool is being applied during their medical care or in research, and about its general workings and the nature of its output. Such transparency is key for making an informed consent and duly exercising ones right to autonomy and bodily integrity.
Some concerns are important as a matter of principle, and should be taken into account in the design and pre-implementation stages of any AI tool, and for AI in healthcare, in particular. Their relevance will be typically manifested in adverse circumstances. Namely, where patients, research participants or medical practitioners implementing the AI tool, seek to legally challenge harmful effects of its operation, or its output.
The following, are such concerns:
- (Non-)explainability of the inner workings of the algorithm of the AI tool, often related to algorithmic opacity.
- Responsibility and accountability gaps – who is accountable for an AI-related harm in healthcare?
Profession-specific (ethical and practical) concerns
- Medical practitioner-in-the-loop? This concern seeks to determine the level of the medical practitioner’s involvement in the decision-making process. Namely, is the AI system a merely decision support tool, designed to assist in medical decision-making, or is it a replacive tool – intended, for example, to provide clinical diagnoses or recommendations in the physician’s stead?
- Physician deskilling. The potentialerosion of physicians’ skills, in the face of smart technology taking over tasks that were typically part of physicians’ near-exclusive expertise and mission, is a tangible concern. Conversely, the potential for augmented intelligence for professionals working alongside intelligent technologies, is often cited when the deskilling argument is invoked.
- Medical education and training challenge. Working alongside AI, underscores the need for a designated training for AI-assisted healthcare professionals. Present-day physicians are largely unequipped to interact with AI-based technologies, in the sense that it was not part of their basic professional training and education. Furthermore, some physicians may be less digitally literate than others. This may require reframing medical education, so that it includes specific attention to AI in healthcare. This includes introduction to AI ethics principles, in the context of medicine.
- Trust building with AI. This concern is relevant for both physicians and patients, and is related to other concerns cited directly above and below, concerning physicians and patients, respectively. Achieving sufficient trust in AI applications in the field of healthcare, is a fundamental challenge to overcome. It will be a shorter process for technology-enthusiasts (and for patients having more confidence in seemingly neutral machines) and a slightly longer one, for technology-sceptics.
- The (altered) nature of physician-patient relationship. The introduction of AI into the clinic, may potentially change the traditional, accustomed interaction between physician and patient. Patient-physician disconnect or alienation, could be one feared outcome (although some AI tools’ goal is freeing up physicians’ time, to allow for a more interpersonal connection between physician and patient). Another set of apprehensions concerns patient scepticism and consequent reduction of trust in physicians and lower treatment compliance.
Let’s not forget about bioethics
Arguably, the deployment of AI in the healthcare field, generates another layer of examination – that of bioethics. That is, the above (non-exhaustive) list of AI-ethics concerns and principles, is supplemented by more traditional bioethical principles, having to do with safeguarding the rights and interests of patients and research participants, in general. That is, ethical protection and guidance designed and shaped long before the nascence of AI-ethics framework. Naturally, there is some overlap between the two sets of principle.
The answer to the question – “when do we then additionally apply bioethics principles?” is context-dependent. Namely, where the application of AI-tools in healthcare stands to meaningfully affect patients/research participants. In such case, due regard must be given to whether, and in what way, their rights to human dignity, autonomy (through transparency and informed consent), beneficence (the usefulness of the tool), and non-maleficence (avoidance of harm), are compromised. Lastly, from a non-personal, societal or community perspectives this may invoke wider concerns for equality (accessibility to benefitting AI tools, including affordability; and relevance of tools trained on (non-) representative data), justice, and fairness (terms/criterions for access).
The validity and persistence of the above-cited ethical dilemmas and practical concerns, largely hinge on time, and on the nature of the experience. Namely: proof of success of the integration of AI into healthcare, against negligent evidence of consequent harm, over a sufficient period of time. Should that be satisfactorily achieved, we shall be able to proclaim that human ingenuity has indeed triumphed through artificial intelligence.