Artificial Intelligence in Healthcare and Social Justice: Barriers and Responses
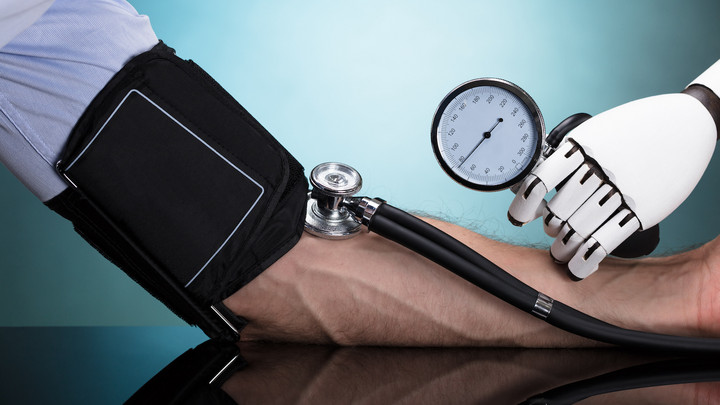
It is universally accepted that everyone has the right to the enjoyment of the highest attainable standard of physical and mental health. The right to health implies various other entitlements, such as the right to a system of health providing equal opportunity for enjoying the highest attainable level of health, the right to prevention, treatment and control of diseases; access to essential treatments and medicines; and equal and timely access to basic health services.
Artificial Intelligence (AI) healthcare technologies are on the brink of becoming the highest standard of health. A wide range of AI-based healthcare technologists is in various stages of implementation: telehealth; tools used for prediction and diagnosis of illness; tools for predicting patient response to treatment; decision support systems; conversational agents and virtual personal health care assistants, and more. Their universal attainability, however, is another matter.
AI healthcare technologies, holding great promise for improved healthcare outcomes, carry a dual (conflicting) potential for narrowing healthcare disparities and, at the same time, exacerbating them.
Reducing healthcare disparities by AI technologies occurs through facilitating healthcare delivery and consumption. Telemedicine is a familiar example. Another, more specific example, is the harnessing of digital health and drones in Northeast India to overcome geographical barriers and improve accessibility to remote, difficult geographical locations due to topographical constraints and poor road connectivity. These can improve access to vaccines and other medical products, as well as to lab samples. AI will be able to take such use of drones beyond proactive home service, to provide personalised healthcare services.
Such technologies, therefore, present an opportunity for population groups typically excluded from medical research or struggling to gain access to conventional healthcare services, to benefit from improved accessible healthcare, thereby somewhat rectifying social injustices. The availability of AI healthcare technologies and their relative ease of accessibility also present a tangible option for democratising healthcare in challenging areas.
On the other hand, by being particularly inaccessible to lower socioeconomic populations due to various inherent barriers described below, such technologies may exacerbate existing social and healthcare disparities and accentuate bias. Furthermore, once becoming more prevalent, offering an alternative to conventional healthcare, AI healthcare technologies may increasingly replace health professionals, leading to the reduction in their number and the deskilling of those still practising. That also stands to adversely affect disadvantaged populations, relying on traditional healthcare. These looming outcomes mandate caution and policy-planning in the widespread application of AI technologies in healthcare.
Barriers to reducing global disparities via AI healthcare technology
From a socio-global perspective, for AI healthcare technology to be effectively harnessed and used in lower socioeconomic populations and developing countries, various barriers need to be primarily overcome. I shall address the following key challenging barriers:
- Health illiteracy
Commonly associated with access to health care and uptake thereof, the right to health extends to include health-related education and information, among other social determinants of health. Health literacy is a complementary, subjective aspect pertaining to the ability to realise one’s right to health in an age of ubiquitous and democratised medical knowledge and empowered, self-health managing patients. It is essentially about people’s capacities, skills, and motivation to understand, access, and apply health information.
A more advanced, digital-leaning version of health literacy concerns the interaction dimension, namely: the ‘individual ability and motivation to engage with digital services and the feeling of being safe and in control of digital technology.’
Health illiteracy is the ‘inability to comprehend and use medical information that can affect access to and use of the healthcare system’ and the processing and application of health information in the context of disease prevention. Such inability can consequently affect the capacity of the health system itself ‘to serve patients and clients.‘ Health illiteracy, associated with overall poorer health, is so widespread and disconcerting that it gained the term ‘the silent epidemic’. Apparently, half of American adults exhibit low health literacy. The European health literacy survey (HLS-EU), conducted in 2011 across eight European countries, found that 12% of respondents have insufficient health literacy, and 47% have limited (insufficient or problematic) health literacy.
In lower socioeconomic populations, digital illiteracy (discussed below) is often accompanied by health illiteracy. The combination of the two seems to yield insecurity and (uninformed) distrust in health information technologies, thus serving as a deterrent to their adoption by those who may stand to profit the most from technological advances.
2. Digital illiteracy and the ‘digital divide’
As our daily lives become ever-increasingly digital, digital literacy – the ability and skills to autonomously and successfully navigate digital environments – becomes a sine-qua-non condition for managing one’s life in the digital and virtual space. This goes beyond the point of personal convenience and social acceptance to the fundamental ability to acquire various services and the realisation of one’s human and civil rights. They all depend on our ability to agreeably interact with digitation.
Digital illiteracy is typically supplemented by (physical and financial) inaccessibility to internet connectivity, information and communication technology infrastructure, and devices – what is dubbed by the World Health Organisation (WHO), the ‘digital divide’.
This is a fundamental barrier to the effective uptake of AI healthcare tools in resource-poor countries and rural areas. The ‘digital divide’ refers to inequitable ‘distribution of access to, use of or effect’ of digital resources among distinct groups. Given the dynamicity of emerging AI healthcare solutions and their inherent personal and public health benefits, the digital divide is not a static, descriptive concept but one carrying the potential to exacerbate existing healthcare inequalities, unless countries take appropriate measures to tackle it. But responsible and ethical governance does not stop here. Technology providers will also be required to play their part in the interest of social justice in healthcare, by providing affordable devices and interoperable infrastructure and services, to allow different platforms/applications to operate seamlessly with one another.
The essentiality of digital literacy in our digital age, and the dependency on such literacy for accessing medical services and monitoring, were greatly felt and emphasised during the COVID-19 pandemic, particularly for the elderly, circumscribed by social isolation.
Medical algorithms can often be plagued with bias historically embedded into the data upon which an algorithm is trained and operated. Oftentimes, it is the non-diversity and misrepresentation of underserved populations in the training set that has a direct bearing on the algorithm’s ability to reduce various unexplained disparities.This, in turn, generates (or rather, reflects) discrimination against particular groups, creates novel health inequalities, or exacerbates existing ones.
An often-cited example is that of a widely deployed algorithm used by health systems to identify patients who would be candidates for ‘high risk-care management’, thus potentially benefiting from special attention. The algorithm, relying on patients’ medical histories and past healthcare expenditure to predict medical risks, exhibited significant racial bias, since racial minorities typically have lower accessibility to healthcare services and spend less money on health care than other social groups. Consideration of actual healthcare expenditure, therefore, failed to authentically reflect these patients’ health risks or status.
Measures to mitigate healthcare disparities via AI healthcare technologies
Digital inclusion is mainly about education in information and communication technologies, and the development of basic ability and set of digital skills to manage one’s health on digital platforms and in other walks of (digitised) life. Where digital exclusion is identified, a potential solution can come in the form of accessible and affordable digital literacy workshops for digitally illiterate populations. Higher rates of digital inclusion will increase potential uptake of AI healthcare technologies, which, in turn, can improve access to healthcare and reduce health inequities.
2. Increasing health data inclusivity
As noted above, health data misrepresentation of marginalized communities, or in other words – individuals who are not Caucasians of European descent, is an infamous fact. Being excluded from health datasets entails the inapplicability of newly developed drugs, therapies and various biomedical technologies for such populations. Inclusion of misrepresented groups and in health (mainly, genetic) databases through research participation and a calculated collection of more representative health data, will ensure that ‘datasets for training and testing AI healthcare technologies are diverse and inclusive.’ The UK, for instance, is set to implement a series of hi-tech initiatives for tackling health disparities among Black, Asian and minority ethnic Britons. One such initiative would be drawing up new standards for health data inclusivity.
3. Correcting Algorithmic discrimination
While (medical) algorithms are typically perceived as a cause of bias, some suggest they can be harnessed to reduce health disparities.Arguably, this can be done by reformulating the algorithm so that it no longer uses bias-inducing data to eliminate bias, or by taking a preemptive approach, e.g., by proactively harnessing algorithms to remedy racial disparities in healthcare. This can broadly include using algorithms to investigate factors behind adverse health outcomes for patients of underserved communities, such as UK Black women’s five-fold higher mortality rate (compared with white women) due to pregnancy-related complications.
Another illustration of the corrective power of algorithms, is the development of an algorithmic approach aimed at reducing unexplained pain disparities in marginalised populations. Such approach can potentially improve prognosis and risk assessment in such populations. While National Institutes of Health data indicates that Black patients and lower-income populations report higher levels of pain, a recent study of an AI system in radiology found that ‘radiologists may have literal blind spots when it comes to reading Black patients’ x-rays.’ That is, they are simply not ‘as proficient in assessing knee pain in Black patients.’ This should come as no surprise, as presently used pain grading is based on ‘a small 1957 study in a northern England mill town with a less diverse population than the modern US.’ The AI in radiology study concluded that algorithms trained on Black patients’ own accounts of pain, rather than mimicking medical experts’ opinions, can promote more equitable healthcare.
On the other hand, a 2020 study published in New England Journal of Medicine has illustrated the potential for race-adjusted algorithms – namely, ‘diagnostic algorithms… that adjust or “correct” their outputs on the basis of a patient’s race or ethnicity’ – to perpetuate or exacerbate race-based health inequities. It was found that many of those algorithms including race in the basic data, guide clinical decisions in ways that may direct more attention or resources to white patients over patients of racial and ethnic minorities. The consideration of race/ethnicity also impairs individualised risk assessment, as such algorithms typically ‘underestimated African Americans’ risks of kidney stones, death from heart failure, and other medical problems.’
One may suggest that the merits of using race-adjusted algorithms are yet to be determined, and until then, they should be evaluated according to circumstance and with great caution.
Conclusion
To conclude, AI healthcare technologies have the power to remedy past wrongs, as well as perpetuate them. From a global perspective, they also carry the potential to democratise healthcare particularly in developing countries, providing that deployment of AI within resource-poor healthcare providers is more equitable. Gradual removal of health and digital literacy barriers and proactive, thoughtful and creative design of medical algorithms, can be valuable in remedying some health inequalities and promoting social health justice amid medically neglected populations.