Taming The Golem: Challenges of Ethical Algorithmic Decision-Making
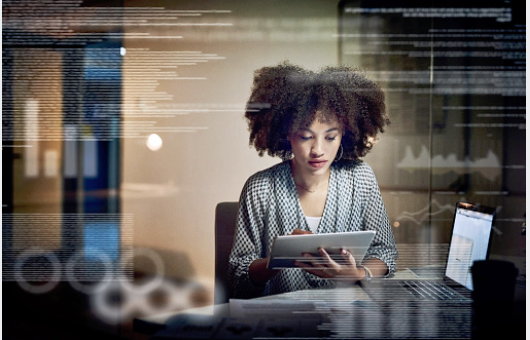
Omer Tene and Jules Polonetsky recently published, “Taming The Golem: Challenges of Ethical Algorithmic Decision-Making,” in Volume 19, Issue 1, of the North Carolina Journal of Law and Technology.
The prospect of digital manipulation on major online platforms reached fever pitch in the last election cycle in the United States. Jonathan Zittrain’s concern about “digital gerrymandering” found resonance in reports, which were resoundingly denied by Facebook, of the company’s alleged editing of content to tone down conservative voices. At the start of the last election cycle, critics blasted Facebook for allegedly injecting editorial bias into an apparently neutral content generator: its “Trending Topics” feature. Immediately after the election when the extent of dissemination of “fake news” through social media became known, commentators chastised Facebook for not proactively policing user- generated content to block and remove untrustworthy information. Which one is it then? Should Facebook have employed policy- directed technologies or should its content algorithm have remained policy-neutral?
This article examines the potential for bias and discrimination in automated algorithmic decision-making. As a group of commentators recently asserted, “[t]he accountability mechanisms and legal standards that govern such decision processes have not kept pace with technology.” Yet this article rejects an approach that depicts every algorithmic process as a “black box” that is inevitably plagued by bias and potential injustice. While recognizing that algorithms are man-made artifacts, written and edited by humans in order to code decision-making processes, the article argues that a distinction should be drawn between “policy-neutral algorithms,” which lack an active editorial hand, and “policy-directed algorithms,” which are intentionally framed to further a designer’s policy agenda.
Policy-neutral algorithms could, in some cases, reflect existing societal biases and historical inequities. Companies, in turn, can choose to fix their results through active social engineering. For example, after facing controversy in light of an algorithmic determination to not offer same-day delivery in low-income neighborhoods, Amazon nevertheless recently decided to provide those services in order to pursue an agenda of equal opportunity. Recognizing that its decision-making process, which was based on logistical factors and expected demand, had the effect of facilitating prevailing social inequality, Amazon chose to level the playing field.
Policy-directed algorithms are purposely engineered to correct for apparent bias and discrimination or to advance a predefined policy agenda. In this case, it is essential that companies provide transparency about their active pursuits of editorial policies. For example, if a search engine decides to scrub results clean of opposing viewpoints, it should let users know they are seeing a manicured version of the world. If a service optimizes results for financial motives without alerting users, it risks violating FTC standards for disclosure. So too should service providers consider themselves obligated to prominently disclose important criteria that reflect an unexpected policy agenda. The transparency called for is not one based on revealing source code but rather public accountability about the editorial nature of the algorithm.
The article addresses questions surrounding the boundaries of responsibility for algorithmic fairness and analyzes a series of case studies under the proposed framework.